Eland 网络研讨会简介#
此 Jupyter 笔记本与 YouTube 上的“Eland 简介”网络研讨会相对应。要进行操作,请在 Elastic Cloud 上创建一个 Elasticsearch 部署(提供免费试用)或在本地启动您自己的 Elasticsearch 集群。
您需要安装以下库
$ python -m pip install eland numpy pandas
DataFrame 演示#
[19]:
# Standard imports
import eland as ed
import pandas as pd
import numpy as np
from elasticsearch import Elasticsearch
# Function for pretty-printing JSON
def json(x):
import json
print(json.dumps(x, indent=2, sort_keys=True))
[20]:
# Connect to an Elastic Cloud instance
# or another Elasticsearch index below
ELASTIC_CLOUD_ID = "<cloud-id>"
ELASTIC_CLOUD_PASSWORD = "<password>"
es = Elasticsearch(
cloud_id=ELASTIC_CLOUD_ID,
basic_auth=("elastic", ELASTIC_CLOUD_PASSWORD)
)
json(es.info())
{
"cluster_name": "167e473c7bba4bae85004385d4e0ce46",
"cluster_uuid": "4Y2FwBhRSsWq9uGedb1DmQ",
"name": "instance-0000000000",
"tagline": "You Know, for Search",
"version": {
"build_date": "2020-06-14T19:35:50.234439Z",
"build_flavor": "default",
"build_hash": "757314695644ea9a1dc2fecd26d1a43856725e65",
"build_snapshot": false,
"build_type": "docker",
"lucene_version": "8.5.1",
"minimum_index_compatibility_version": "6.0.0-beta1",
"minimum_wire_compatibility_version": "6.8.0",
"number": "7.8.0"
}
}
[21]:
# Load the dataset from NYC Open Data and take a look
pd_df = pd.read_csv("nyc-restaurants.csv").dropna()
pd_df.info()
<class 'pandas.core.frame.DataFrame'>
Int64Index: 193197 entries, 0 to 400255
Data columns (total 26 columns):
# Column Non-Null Count Dtype
--- ------ -------------- -----
0 CAMIS 193197 non-null int64
1 DBA 193197 non-null object
2 BORO 193197 non-null object
3 BUILDING 193197 non-null object
4 STREET 193197 non-null object
5 ZIPCODE 193197 non-null float64
6 PHONE 193197 non-null object
7 CUISINE DESCRIPTION 193197 non-null object
8 INSPECTION DATE 193197 non-null object
9 ACTION 193197 non-null object
10 VIOLATION CODE 193197 non-null object
11 VIOLATION DESCRIPTION 193197 non-null object
12 CRITICAL FLAG 193197 non-null object
13 SCORE 193197 non-null float64
14 GRADE 193197 non-null object
15 GRADE DATE 193197 non-null object
16 RECORD DATE 193197 non-null object
17 INSPECTION TYPE 193197 non-null object
18 Latitude 193197 non-null float64
19 Longitude 193197 non-null float64
20 Community Board 193197 non-null float64
21 Council District 193197 non-null float64
22 Census Tract 193197 non-null float64
23 BIN 193197 non-null float64
24 BBL 193197 non-null float64
25 NTA 193197 non-null object
dtypes: float64(9), int64(1), object(16)
memory usage: 39.8+ MB
[22]:
# Rename the columns to be snake_case
pd_df.columns = [x.lower().replace(" ", "_") for x in pd_df.columns]
# Combine the 'latitude' and 'longitude' columns into one column 'location' for 'geo_point'
pd_df["location"] = pd_df[["latitude", "longitude"]].apply(lambda x: ",".join(str(item) for item in x), axis=1)
# Drop the old columns in favor of 'location'
pd_df.drop(["latitude", "longitude"], axis=1, inplace=True)
pd_df.info()
<class 'pandas.core.frame.DataFrame'>
Int64Index: 193197 entries, 0 to 400255
Data columns (total 25 columns):
# Column Non-Null Count Dtype
--- ------ -------------- -----
0 camis 193197 non-null int64
1 dba 193197 non-null object
2 boro 193197 non-null object
3 building 193197 non-null object
4 street 193197 non-null object
5 zipcode 193197 non-null float64
6 phone 193197 non-null object
7 cuisine_description 193197 non-null object
8 inspection_date 193197 non-null object
9 action 193197 non-null object
10 violation_code 193197 non-null object
11 violation_description 193197 non-null object
12 critical_flag 193197 non-null object
13 score 193197 non-null float64
14 grade 193197 non-null object
15 grade_date 193197 non-null object
16 record_date 193197 non-null object
17 inspection_type 193197 non-null object
18 community_board 193197 non-null float64
19 council_district 193197 non-null float64
20 census_tract 193197 non-null float64
21 bin 193197 non-null float64
22 bbl 193197 non-null float64
23 nta 193197 non-null object
24 location 193197 non-null object
dtypes: float64(7), int64(1), object(17)
memory usage: 38.3+ MB
[23]:
df = ed.pandas_to_eland(
pd_df=pd_df,
es_client=es,
# Where the data will live in Elasticsearch
es_dest_index="nyc-restaurants",
# Type overrides for certain columns, 'location' detected
# automatically as 'keyword' but we want these interpreted as 'geo_point'.
es_type_overrides={
"location": "geo_point",
"dba": "text",
"zipcode": "short"
},
# If the index already exists what should we do?
es_if_exists="replace",
# Wait for data to be indexed before returning
es_refresh=True,
)
df.info()
<class 'eland.dataframe.DataFrame'>
Index: 193197 entries, 10388 to 398749
Data columns (total 25 columns):
# Column Non-Null Count Dtype
--- ------ -------------- -----
0 camis 193197 non-null int64
1 dba 193197 non-null object
2 boro 193197 non-null object
3 building 193197 non-null object
4 street 193197 non-null object
5 zipcode 193197 non-null int64
6 phone 193197 non-null object
7 cuisine_description 193197 non-null object
8 inspection_date 193197 non-null object
9 action 193197 non-null object
10 violation_code 193197 non-null object
11 violation_description 193197 non-null object
12 critical_flag 193197 non-null object
13 score 193197 non-null float64
14 grade 193197 non-null object
15 grade_date 193197 non-null object
16 record_date 193197 non-null object
17 inspection_type 193197 non-null object
18 community_board 193197 non-null float64
19 council_district 193197 non-null float64
20 census_tract 193197 non-null float64
21 bin 193197 non-null float64
22 bbl 193197 non-null float64
23 nta 193197 non-null object
24 location 193197 non-null object
dtypes: float64(6), int64(2), object(17)
memory usage: 80.0 bytes
[24]:
json(es.indices.get_mapping(index="nyc-restaurants"))
{
"nyc-restaurants": {
"mappings": {
"properties": {
"action": {
"type": "keyword"
},
"bbl": {
"type": "double"
},
"bin": {
"type": "double"
},
"boro": {
"type": "keyword"
},
"building": {
"type": "keyword"
},
"camis": {
"type": "long"
},
"census_tract": {
"type": "double"
},
"community_board": {
"type": "double"
},
"council_district": {
"type": "double"
},
"critical_flag": {
"type": "keyword"
},
"cuisine_description": {
"type": "keyword"
},
"dba": {
"type": "text"
},
"grade": {
"type": "keyword"
},
"grade_date": {
"type": "keyword"
},
"inspection_date": {
"type": "keyword"
},
"inspection_type": {
"type": "keyword"
},
"location": {
"type": "geo_point"
},
"nta": {
"type": "keyword"
},
"phone": {
"type": "keyword"
},
"record_date": {
"type": "keyword"
},
"score": {
"type": "double"
},
"street": {
"type": "keyword"
},
"violation_code": {
"type": "keyword"
},
"violation_description": {
"type": "keyword"
},
"zipcode": {
"type": "short"
}
}
}
}
}
[25]:
# Shape is determined by using count API
df.shape
[25]:
(193197, 25)
[34]:
# DataFrame has many APIs compatible with Pandas
#df.head(10)
#df.columns
#df.dba
#df["grade"]
#df[df.grade.isin(["A", "B"])]
#print(df[df.grade.isin(["A", "B"])].es_info())
#print(df.tail(10).es_info())
es_index_pattern: nyc-restaurants
Index:
es_index_field: _id
is_source_field: False
Mappings:
capabilities:
es_field_name is_source es_dtype es_date_format pd_dtype is_searchable is_aggregatable is_scripted aggregatable_es_field_name
camis camis True long None int64 True True False camis
dba dba True text None object True False False None
boro boro True keyword None object True True False boro
building building True keyword None object True True False building
street street True keyword None object True True False street
zipcode zipcode True short None int64 True True False zipcode
phone phone True keyword None object True True False phone
cuisine_description cuisine_description True keyword None object True True False cuisine_description
inspection_date inspection_date True keyword None object True True False inspection_date
action action True keyword None object True True False action
violation_code violation_code True keyword None object True True False violation_code
violation_description violation_description True keyword None object True True False violation_description
critical_flag critical_flag True keyword None object True True False critical_flag
score score True double None float64 True True False score
grade grade True keyword None object True True False grade
grade_date grade_date True keyword None object True True False grade_date
record_date record_date True keyword None object True True False record_date
inspection_type inspection_type True keyword None object True True False inspection_type
community_board community_board True double None float64 True True False community_board
council_district council_district True double None float64 True True False council_district
census_tract census_tract True double None float64 True True False census_tract
bin bin True double None float64 True True False bin
bbl bbl True double None float64 True True False bbl
nta nta True keyword None object True True False nta
location location True geo_point None object True True False location
Operations:
tasks: [('tail': ('sort_field': '_doc', 'count': 10))]
size: 10
sort_params: _doc:desc
_source: ['camis', 'dba', 'boro', 'building', 'street', 'zipcode', 'phone', 'cuisine_description', 'inspection_date', 'action', 'violation_code', 'violation_description', 'critical_flag', 'score', 'grade', 'grade_date', 'record_date', 'inspection_type', 'community_board', 'council_district', 'census_tract', 'bin', 'bbl', 'nta', 'location']
body: {}
post_processing: [('sort_index')]
[39]:
# Aggregating values
df.describe()
[39]:
camis | zipcode | score | community_board | council_district | census_tract | bin | bbl | |
---|---|---|---|---|---|---|---|---|
count | 1.931970e+05 | 193197.000000 | 193197.000000 | 193197.000000 | 193197.000000 | 193197.000000 | 1.931970e+05 | 1.931970e+05 |
mean | 4.605010e+07 | 10677.212540 | 12.947680 | 248.602603 | 20.020715 | 28796.048298 | 2.513373e+06 | 2.450622e+09 |
std | 4.415232e+06 | 595.142246 | 8.180244 | 130.697014 | 15.809664 | 30672.683469 | 1.351134e+06 | 1.313578e+09 |
min | 3.011234e+07 | 10000.000000 | -1.000000 | 101.000000 | 1.000000 | 100.000000 | 1.000000e+06 | 1.000000e+09 |
25% | 4.138051e+07 | 10022.000000 | 9.000000 | 105.000000 | 4.000000 | 7895.605691 | 1.042708e+06 | 1.011024e+09 |
50% | 5.000527e+07 | 10468.006114 | 12.000000 | 301.000000 | 19.747529 | 16022.917106 | 3.007191e+06 | 3.002924e+09 |
75% | 5.005661e+07 | 11228.624535 | 13.000000 | 401.000000 | 34.000000 | 40246.000337 | 4.002294e+06 | 4.003343e+09 |
max | 5.010416e+07 | 12345.000000 | 99.000000 | 503.000000 | 51.000000 | 162100.000000 | 5.799501e+06 | 5.270001e+09 |
[40]:
# Plotting with matplotlib
from matplotlib import pyplot as plt
df[["score"]].hist(figsize=[10,10])
plt.show()
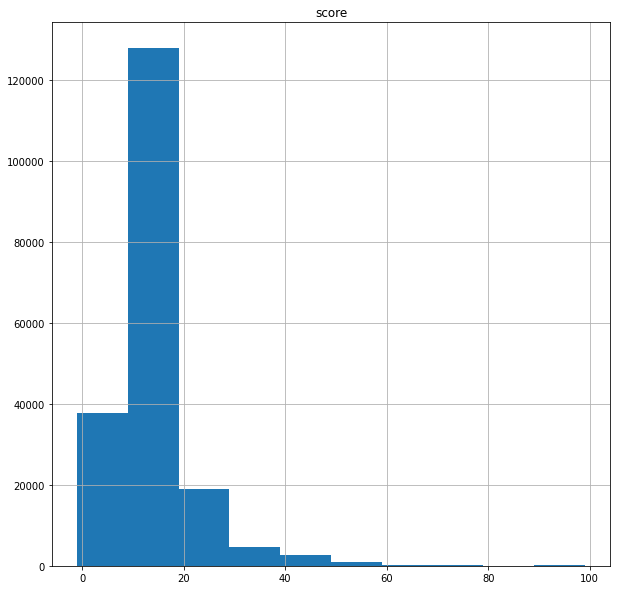
[42]:
# es_query() allows for the full Elasticsearch querying capabilities
df.es_query({
"geo_distance": {
"distance": "50m",
"location": {
"lat": 40.643852716573,
"lon": -74.011628212186
}
}
})
[42]:
camis | dba | boro | building | street | zipcode | phone | cuisine_description | inspection_date | action | ... | grade_date | record_date | inspection_type | community_board | council_district | census_tract | bin | bbl | nta | location | |
---|---|---|---|---|---|---|---|---|---|---|---|---|---|---|---|---|---|---|---|---|---|
53127 | 41144258 | BURGER KING | 布鲁克林 | 5212 | 5 AVENUE | 11220.0 | 7187650844 | 汉堡包 | 12/26/2018 | 以下区域存在违规行为。 | ... | 12/26/2018 | 07/07/2020 | 循环检查/初始检查 | 307.0 | 38.0 | 7600.0 | 3329902.0 | 3.008070e+09 | BK32 | 40.643852716573,-74.011628212186 |
61268 | 41144258 | BURGER KING | 布鲁克林 | 5212 | 5 AVENUE | 11220.0 | 7187650844 | 汉堡包 | 07/20/2017 | 以下区域存在违规行为。 | ... | 07/20/2017 | 07/07/2020 | 循环检查/初始检查 | 307.0 | 38.0 | 7600.0 | 3329902.0 | 3.008070e+09 | BK32 | 40.643852716573,-74.011628212186 |
20717 | 41144258 | BURGER KING | 布鲁克林 | 5212 | 5 AVENUE | 11220.0 | 7187650844 | 汉堡包 | 03/04/2020 | 以下区域存在违规行为。 | ... | 03/04/2020 | 07/07/2020 | 循环检查/初始检查 | 307.0 | 38.0 | 7600.0 | 3329902.0 | 3.008070e+09 | BK32 | 40.643852716573,-74.011628212186 |
4648 | 41271801 | PINO'S | 布鲁克林 | 5201 | 5 AVENUE | 11220.0 | 7184396012 | 比萨饼 | 05/25/2019 | 以下区域存在违规行为。 | ... | 05/25/2019 | 07/07/2020 | 循环检查/初始检查 | 307.0 | 38.0 | 10000.0 | 3013942.0 | 3.008080e+09 | BK34 | 40.643888405293005,-74.011563356969 |
224 | 41271801 | PINO'S | 布鲁克林 | 5201 | 5 AVENUE | 11220.0 | 7184396012 | 比萨饼 | 05/25/2019 | 以下区域存在违规行为。 | ... | 05/25/2019 | 07/07/2020 | 循环检查/初始检查 | 307.0 | 38.0 | 10000.0 | 3013942.0 | 3.008080e+09 | BK34 | 40.643888405293005,-74.011563356969 |
9465 | 41144258 | BURGER KING | 布鲁克林 | 5212 | 5 AVENUE | 11220.0 | 7187650844 | 汉堡包 | 03/04/2020 | 以下区域存在违规行为。 | ... | 03/04/2020 | 07/07/2020 | 循环检查/初始检查 | 307.0 | 38.0 | 7600.0 | 3329902.0 | 3.008070e+09 | BK32 | 40.643852716573,-74.011628212186 |
104512 | 40396492 | ROYAL KING'S PIZZA | 布鲁克林 | 5211 | 5 AVENUE | 11220.0 | 7184923846 | 比萨饼 | 12/19/2018 | 以下区域存在违规行为。 | ... | 12/19/2018 | 07/07/2020 | 循环检查/初始检查 | 307.0 | 38.0 | 10000.0 | 3013939.0 | 3.008080e+09 | BK34 | 40.643849974348996,-74.01160298782 |
106728 | 41271801 | PINO'S | 布鲁克林 | 5201 | 5 AVENUE | 11220.0 | 7184396012 | 比萨饼 | 01/25/2018 | 以下区域存在违规行为。 | ... | 01/25/2018 | 07/07/2020 | 循环检查/复查 | 307.0 | 38.0 | 10000.0 | 3013942.0 | 3.008080e+09 | BK34 | 40.643888405293005,-74.011563356969 |
62748 | 50004330 | 肯德基 | 布鲁克林 | 5223 | 5 AVENUE | 11220.0 | 7184922813 | 鸡肉 | 05/28/2019 | 以下区域存在违规行为。 | ... | 05/28/2019 | 07/07/2020 | 循环检查/初始检查 | 307.0 | 38.0 | 10000.0 | 3013937.0 | 3.008080e+09 | BK34 | 40.643800563168,-74.01165342693001 |
79211 | 41271801 | PINO'S | 布鲁克林 | 5201 | 5 AVENUE | 11220.0 | 7184396012 | 比萨饼 | 11/05/2016 | 以下区域存在违规行为。 | ... | 11/05/2016 | 07/07/2020 | 循环检查/初始检查 | 307.0 | 38.0 | 10000.0 | 3013942.0 | 3.008080e+09 | BK34 | 40.643888405293005,-74.011563356969 |
218545 | 50004330 | 肯德基 | 布鲁克林 | 5223 | 5 AVENUE | 11220.0 | 7184922813 | 鸡肉 | 01/10/2018 | 以下区域存在违规行为。 | ... | 01/10/2018 | 07/07/2020 | 循环检查/复查 | 307.0 | 38.0 | 10000.0 | 3013937.0 | 3.008080e+09 | BK34 | 40.643800563168,-74.01165342693001 |
238663 | 41271801 | PINO'S | 布鲁克林 | 5201 | 5 AVENUE | 11220.0 | 7184396012 | 比萨饼 | 11/05/2016 | 以下区域存在违规行为。 | ... | 11/05/2016 | 07/07/2020 | 循环检查/初始检查 | 307.0 | 38.0 | 10000.0 | 3013942.0 | 3.008080e+09 | BK34 | 40.643888405293005,-74.011563356969 |
245205 | 40396492 | ROYAL KING'S PIZZA | 布鲁克林 | 5211 | 5 AVENUE | 11220.0 | 7184923846 | 比萨饼 | 12/19/2018 | 以下区域存在违规行为。 | ... | 12/19/2018 | 07/07/2020 | 循环检查/初始检查 | 307.0 | 38.0 | 10000.0 | 3013939.0 | 3.008080e+09 | BK34 | 40.643849974348996,-74.01160298782 |
245233 | 41271801 | PINO'S | 布鲁克林 | 5201 | 5 AVENUE | 11220.0 | 7184396012 | 比萨饼 | 01/25/2018 | 以下区域存在违规行为。 | ... | 01/25/2018 | 07/07/2020 | 循环检查/复查 | 307.0 | 38.0 | 10000.0 | 3013942.0 | 3.008080e+09 | BK34 | 40.643888405293005,-74.011563356969 |
247417 | 50004330 | 肯德基 | 布鲁克林 | 5223 | 5 AVENUE | 11220.0 | 7184922813 | 鸡肉 | 05/05/2017 | 以下区域存在违规行为。 | ... | 05/05/2017 | 07/07/2020 | 循环检查/复查 | 307.0 | 38.0 | 10000.0 | 3013937.0 | 3.008080e+09 | BK34 | 40.643800563168,-74.01165342693001 |
186874 | 50099704 | MASTER'S PIZZERIA | 布鲁克林 | 5201 | 5 AVENUE | 11220.0 | 7184396012 | 比萨饼 | 11/18/2019 | 以下区域存在违规行为。 | ... | 11/18/2019 | 07/07/2020 | 预许可(运营)/初始检查 | 307.0 | 38.0 | 10000.0 | 3013942.0 | 3.008080e+09 | BK34 | 40.643888405293005,-74.011563356969 |
198104 | 40396492 | ROYAL KING'S PIZZA | 布鲁克林 | 5211 | 5 AVENUE | 11220.0 | 7184923846 | 比萨饼 | 12/28/2017 | 以下区域存在违规行为。 | ... | 12/28/2017 | 07/07/2020 | 循环检查/初始检查 | 307.0 | 38.0 | 10000.0 | 3013939.0 | 3.008080e+09 | BK34 | 40.643849974348996,-74.01160298782 |
213425 | 40396492 | ROYAL KING'S PIZZA | 布鲁克林 | 5211 | 5 AVENUE | 11220.0 | 7184923846 | 比萨饼 | 12/19/2018 | 以下区域存在违规行为。 | ... | 12/19/2018 | 07/07/2020 | 循环检查/初始检查 | 307.0 | 38.0 | 10000.0 | 3013939.0 | 3.008080e+09 | BK34 | 40.643849974348996,-74.01160298782 |
202363 | 50004330 | 肯德基 | 布鲁克林 | 5223 | 5 AVENUE | 11220.0 | 7184922813 | 鸡肉 | 05/28/2019 | 以下区域存在违规行为。 | ... | 05/28/2019 | 07/07/2020 | 循环检查/初始检查 | 307.0 | 38.0 | 10000.0 | 3013937.0 | 3.008080e+09 | BK34 | 40.643800563168,-74.01165342693001 |
158059 | 40396492 | ROYAL KING'S PIZZA | 布鲁克林 | 5211 | 5 AVENUE | 11220.0 | 7184923846 | 比萨饼 | 12/19/2018 | 以下区域存在违规行为。 | ... | 12/19/2018 | 07/07/2020 | 循环检查/初始检查 | 307.0 | 38.0 | 10000.0 | 3013939.0 | 3.008080e+09 | BK34 | 40.643849974348996,-74.01160298782 |
163672 | 41144258 | BURGER KING | 布鲁克林 | 5212 | 5 AVENUE | 11220.0 | 7187650844 | 汉堡包 | 08/13/2018 | 以下区域存在违规行为。 | ... | 08/13/2018 | 07/07/2020 | 循环检查/复查 | 307.0 | 38.0 | 7600.0 | 3329902.0 | 3.008070e+09 | BK32 | 40.643852716573,-74.011628212186 |
138508 | 40396492 | ROYAL KING'S PIZZA | 布鲁克林 | 5211 | 5 AVENUE | 11220.0 | 7184923846 | 比萨饼 | 01/29/2020 | 以下区域存在违规行为。 | ... | 01/29/2020 | 07/07/2020 | 循环检查/复查 | 307.0 | 38.0 | 10000.0 | 3013939.0 | 3.008080e+09 | BK34 | 40.643849974348996,-74.01160298782 |
140940 | 41144258 | BURGER KING | 布鲁克林 | 5212 | 5 AVENUE | 11220.0 | 7187650844 | 汉堡包 | 07/20/2017 | 以下区域存在违规行为。 | ... | 07/20/2017 | 07/07/2020 | 循环检查/初始检查 | 307.0 | 38.0 | 7600.0 | 3329902.0 | 3.008070e+09 | BK32 | 40.643852716573,-74.011628212186 |
143157 | 50004330 | 肯德基 | 布鲁克林 | 5223 | 5 AVENUE | 11220.0 | 7184922813 | 鸡肉 | 01/10/2018 | 以下区域存在违规行为。 | ... | 01/10/2018 | 07/07/2020 | 循环检查/复查 | 307.0 | 38.0 | 10000.0 | 3013937.0 | 3.008080e+09 | BK34 | 40.643800563168,-74.01165342693001 |
149548 | 41144258 | BURGER KING | 布鲁克林 | 5212 | 5 AVENUE | 11220.0 | 7187650844 | 汉堡包 | 07/20/2017 | 以下区域存在违规行为。 | ... | 07/20/2017 | 07/07/2020 | 循环检查/初始检查 | 307.0 | 38.0 | 7600.0 | 3329902.0 | 3.008070e+09 | BK32 | 40.643852716573,-74.011628212186 |
149742 | 50004330 | 肯德基 | 布鲁克林 | 5223 | 5 AVENUE | 11220.0 | 7184922813 | 鸡肉 | 05/31/2018 | 以下区域存在违规行为。 | ... | 05/31/2018 | 07/07/2020 | 循环检查/初始检查 | 307.0 | 38.0 | 10000.0 | 3013937.0 | 3.008080e+09 | BK34 | 40.643800563168,-74.01165342693001 |
249994 | 41271801 | PINO'S | 布鲁克林 | 5201 | 5 AVENUE | 11220.0 | 7184396012 | 比萨饼 | 01/25/2018 | 以下区域存在违规行为。 | ... | 01/25/2018 | 07/07/2020 | 循环检查/复查 | 307.0 | 38.0 | 10000.0 | 3013942.0 | 3.008080e+09 | BK34 | 40.643888405293005,-74.011563356969 |
257603 | 41144258 | BURGER KING | 布鲁克林 | 5212 | 5 AVENUE | 11220.0 | 7187650844 | 汉堡包 | 08/13/2018 | 以下区域存在违规行为。 | ... | 08/13/2018 | 07/07/2020 | 循环检查/复查 | 307.0 | 38.0 | 7600.0 | 3329902.0 | 3.008070e+09 | BK32 | 40.643852716573,-74.011628212186 |
268823 | 50004330 | 肯德基 | 布鲁克林 | 5223 | 5 AVENUE | 11220.0 | 7184922813 | 鸡肉 | 01/10/2018 | 以下区域存在违规行为。 | ... | 01/10/2018 | 07/07/2020 | 循环检查/复查 | 307.0 | 38.0 | 10000.0 | 3013937.0 | 3.008080e+09 | BK34 | 40.643800563168,-74.01165342693001 |
269521 | 41144258 | BURGER KING | 布鲁克林 | 5212 | 5 AVENUE | 11220.0 | 7187650844 | 汉堡包 | 12/17/2019 | 以下区域存在违规行为。 | ... | 12/17/2019 | 07/07/2020 | 循环检查/复查 | 307.0 | 38.0 | 7600.0 | 3329902.0 | 3.008070e+09 | BK32 | 40.643852716573,-74.011628212186 |
277500 | 50099704 | MASTER'S PIZZERIA | 布鲁克林 | 5201 | 5 AVENUE | 11220.0 | 7184396012 | 比萨饼 | 11/18/2019 | 以下区域存在违规行为。 | ... | 11/18/2019 | 07/07/2020 | 预许可(运营)/初始检查 | 307.0 | 38.0 | 10000.0 | 3013942.0 | 3.008080e+09 | BK34 | 40.643888405293005,-74.011563356969 |
279503 | 40396492 | ROYAL KING'S PIZZA | 布鲁克林 | 5211 | 5 AVENUE | 11220.0 | 7184923846 | 比萨饼 | 01/29/2020 | 以下区域存在违规行为。 | ... | 01/29/2020 | 07/07/2020 | 循环检查/复查 | 307.0 | 38.0 | 10000.0 | 3013939.0 | 3.008080e+09 | BK34 | 40.643849974348996,-74.01160298782 |
299863 | 41144258 | BURGER KING | 布鲁克林 | 5212 | 5 AVENUE | 11220.0 | 7187650844 | 汉堡包 | 12/26/2018 | 以下区域存在违规行为。 | ... | 12/26/2018 | 07/07/2020 | 循环检查/初始检查 | 307.0 | 38.0 | 7600.0 | 3329902.0 | 3.008070e+09 | BK32 | 40.643852716573,-74.011628212186 |
319787 | 41271801 | PINO'S | 布鲁克林 | 5201 | 5 AVENUE | 11220.0 | 7184396012 | 比萨饼 | 05/25/2019 | 以下区域存在违规行为。 | ... | 05/25/2019 | 07/07/2020 | 循环检查/初始检查 | 307.0 | 38.0 | 10000.0 | 3013942.0 | 3.008080e+09 | BK34 | 40.643888405293005,-74.011563356969 |
336570 | 50004330 | 肯德基 | 布鲁克林 | 5223 | 5 AVENUE | 11220.0 | 7184922813 | 鸡肉 | 01/10/2018 | 以下区域存在违规行为。 | ... | 01/10/2018 | 07/07/2020 | 循环检查/复查 | 307.0 | 38.0 | 10000.0 | 3013937.0 | 3.008080e+09 | BK34 | 40.643800563168,-74.01165342693001 |
340551 | 50004330 | 肯德基 | 布鲁克林 | 5223 | 5 AVENUE | 11220.0 | 7184922813 | 鸡肉 | 04/10/2017 | 机构重新开业 | ... | 04/10/2017 | 07/07/2020 | 循环检查/重新开业检查 | 307.0 | 38.0 | 10000.0 | 3013937.0 | 3.008080e+09 | BK34 | 40.643800563168,-74.01165342693001 |
395508 | 41144258 | BURGER KING | 布鲁克林 | 5212 | 5 AVENUE | 11220.0 | 7187650844 | 汉堡包 | 12/17/2019 | 以下区域存在违规行为。 | ... | 12/17/2019 | 07/07/2020 | 循环检查/复查 | 307.0 | 38.0 | 7600.0 | 3329902.0 | 3.008070e+09 | BK32 | 40.643852716573,-74.011628212186 |
309366 | 40396492 | ROYAL KING'S PIZZA | 布鲁克林 | 5211 | 5 AVENUE | 11220.0 | 7184923846 | 比萨饼 | 12/28/2017 | 以下区域存在违规行为。 | ... | 12/28/2017 | 07/07/2020 | 循环检查/初始检查 | 307.0 | 38.0 | 10000.0 | 3013939.0 | 3.008080e+09 | BK34 | 40.643849974348996,-74.01160298782 |
340857 | 40396492 | ROYAL KING'S PIZZA | 布鲁克林 | 5211 | 5 AVENUE | 11220.0 | 7184923846 | 比萨饼 | 01/29/2020 | 以下区域存在违规行为。 | ... | 01/29/2020 | 07/07/2020 | 循环检查/复查 | 307.0 | 38.0 | 10000.0 | 3013939.0 | 3.008080e+09 | BK34 | 40.643849974348996,-74.01160298782 |
358660 | 50004330 | 肯德基 | 布鲁克林 | 5223 | 5 AVENUE | 11220.0 | 7184922813 | 鸡肉 | 05/31/2018 | 以下区域存在违规行为。 | ... | 05/31/2018 | 07/07/2020 | 循环检查/初始检查 | 307.0 | 38.0 | 10000.0 | 3013937.0 | 3.008080e+09 | BK34 | 40.643800563168,-74.01165342693001 |
393451 | 41271801 | PINO'S | 布鲁克林 | 5201 | 5 AVENUE | 11220.0 | 7184396012 | 比萨饼 | 06/05/2018 | 以下区域存在违规行为。 | ... | 06/05/2018 | 07/07/2020 | 循环检查/初始检查 | 307.0 | 38.0 | 10000.0 | 3013942.0 | 3.008080e+09 | BK34 | 40.643888405293005,-74.011563356969 |
41 行 × 25 列
[43]:
# Full-text search example
df.es_query({"match": {"dba": "red"}})
[43]:
camis | dba | boro | building | street | zipcode | phone | cuisine_description | inspection_date | action | ... | grade_date | record_date | inspection_type | community_board | council_district | census_tract | bin | bbl | nta | location | |
---|---|---|---|---|---|---|---|---|---|---|---|---|---|---|---|---|---|---|---|---|---|
5765 | 50033781 | RED HOOK LOBSTER POUND | 布鲁克林 | 284 | VAN BRUNT STREET | 11231.0 | 7188587650 | 海鲜 | 04/19/2018 | 以下区域存在违规行为。 | ... | 04/19/2018 | 07/07/2020 | 循环检查/初始检查 | 306.0 | 38.0 | 5900.0 | 3008365.0 | 3.005290e+09 | BK33 | 40.67974632809,-74.010098611838 |
12379 | 50058053 | RED HOT II | 布鲁克林 | 349 | 7 AVENUE | 11215.0 | 7183692577 | 中国菜 | 05/17/2018 | 以下区域存在违规行为。 | ... | 05/17/2018 | 07/07/2020 | 循环检查/复查 | 306.0 | 39.0 | 15100.0 | 3026127.0 | 3.010940e+09 | BK37 | 40.666194419994,-73.98214269199799 |
12978 | 50059700 | RED POKE | 曼哈顿 | 600 | 9 AVENUE | 10036.0 | 2129748100 | 夏威夷菜 | 03/21/2017 | 以下区域存在违规行为。 | ... | 03/21/2017 | 07/07/2020 | 预许可(运营)/复查 | 104.0 | 3.0 | 12100.0 | 1088997.0 | 1.010330e+09 | MN15 | 40.758993434643,-73.992203122611 |
16759 | 40365239 | DORRIAN'S RED HAND RESTAURANT | 曼哈顿 | 1616 | 2 AVENUE | 10028.0 | 2127726660 | 爱尔兰菜 | 11/08/2018 | 以下区域存在违规行为。 | ... | 11/08/2018 | 07/07/2020 | 循环检查/初始检查 | 108.0 | 5.0 | 13800.0 | 1049947.0 | 1.015460e+09 | MN32 | 40.776404966262,-73.952802065662 |
18624 | 50095340 | RED PEONY CHINESE CUISINE | 曼哈顿 | 24 | WEST 56 STREET | 10019.0 | 2123808883 | 中国菜 | 11/21/2019 | 以下区域存在违规行为。 | ... | 11/21/2019 | 07/07/2020 | 预许可(运营)/复查 | 105.0 | 4.0 | 10400.0 | 1034840.0 | 1.012710e+09 | MN17 | 40.762699245064,-73.975463733228 |
... | ... | ... | ... | ... | ... | ... | ... | ... | ... | ... | ... | ... | ... | ... | ... | ... | ... | ... | ... | ... | ... |
391229 | 50061162 | CODE RED | 布朗克斯 | 1320 | EAST GUN HILL ROAD | 10469.0 | 7188811808 | 加勒比海菜 | 05/14/2018 | 以下区域存在违规行为。 | ... | 05/14/2018 | 07/07/2020 | 循环检查/初始检查 | 211.0 | 12.0 | 35000.0 | 2056100.0 | 2.045890e+09 | BX31 | 40.871378316318996,-73.848028279305 |
393531 | 50014078 | RED LOBSTER | 曼哈顿 | 5 | 时代广场 | 10036.0 | 2127306706 | 海鲜 | 11/08/2017 | 以下区域存在违规行为。 | ... | 11/08/2017 | 07/07/2020 | 循环检查/复查 | 105.0 | 3.0 | 11300.0 | 1024656.0 | 1.010130e+09 | MN17 | 40.755702020307005,-73.987207980138 |
396171 | 40368313 | RED FLAME DINER | 曼哈顿 | 67 | WEST 44 STREET | 10036.0 | 2128693965 | 美式 | 02/16/2018 | 以下区域存在违规行为。 | ... | 02/16/2018 | 07/07/2020 | 循环检查/初始检查 | 105.0 | 4.0 | 9600.0 | 1034217.0 | 1.012600e+09 | MN17 | 40.755627203336,-73.981938150269 |
396501 | 50068499 | RED GINGER | 史坦顿岛 | 1650 | RICHMOND AVENUE | 10314.0 | 7189828808 | 其他 | 09/19/2017 | 以下区域存在违规行为。 | ... | 09/19/2017 | 07/07/2020 | 预许可(运营)/初始检查 | 502.0 | 50.0 | 29103.0 | 5037014.0 | 5.022360e+09 | SI05 | 40.608078102502,-74.162260908042 |
398950 | 50059700 | RED POKE | 曼哈顿 | 600 | 9 AVENUE | 10036.0 | 2129748100 | 夏威夷菜 | 12/08/2017 | 以下区域存在违规行为。 | ... | 12/08/2017 | 07/07/2020 | 循环检查/复查 | 104.0 | 3.0 | 12100.0 | 1088997.0 | 1.010330e+09 | MN15 | 40.758993434643,-73.992203122611 |
573 行 × 25 列
[44]:
# Pull a subset of your data for building graphs / operations locally.
sample_df = df[df.grade == "B"].sample(100).to_pandas()
sample_df.info()
print(type(sample_df))
<class 'pandas.core.frame.DataFrame'>
Index: 100 entries, 107677 to 96813
Data columns (total 25 columns):
# Column Non-Null Count Dtype
--- ------ -------------- -----
0 camis 100 non-null int64
1 dba 100 non-null object
2 boro 100 non-null object
3 building 100 non-null object
4 street 100 non-null object
5 zipcode 100 non-null float64
6 phone 100 non-null object
7 cuisine_description 100 non-null object
8 inspection_date 100 non-null object
9 action 100 non-null object
10 violation_code 100 non-null object
11 violation_description 100 non-null object
12 critical_flag 100 non-null object
13 score 100 non-null float64
14 grade 100 non-null object
15 grade_date 100 non-null object
16 record_date 100 non-null object
17 inspection_type 100 non-null object
18 community_board 100 non-null float64
19 council_district 100 non-null float64
20 census_tract 100 non-null float64
21 bin 100 non-null float64
22 bbl 100 non-null float64
23 nta 100 non-null object
24 location 100 non-null object
dtypes: float64(7), int64(1), object(17)
memory usage: 20.3+ KB
<class 'pandas.core.frame.DataFrame'>
机器学习演示#
[45]:
# Import scikit-learn and train a dataset locally
from sklearn import datasets
from sklearn.tree import DecisionTreeClassifier
# Train the data locally
digits = datasets.load_wine()
print("Feature Names:", digits.feature_names)
print("Data example:", digits.data[0])
# Save 10, 80, and 140 for testing our model
data = [x for i, x in enumerate(digits.data) if i not in (10, 80, 140)]
target = [x for i, x in enumerate(digits.target) if i not in (10, 80, 140)]
sk_classifier = DecisionTreeClassifier()
sk_classifier.fit(data, target)
# Test out our model against the three targets
print(sk_classifier.predict(digits.data[[10, 80, 140]]))
print(digits.target[[10, 80, 140]])
Feature Names: ['alcohol', 'malic_acid', 'ash', 'alcalinity_of_ash', 'magnesium', 'total_phenols', 'flavanoids', 'nonflavanoid_phenols', 'proanthocyanins', 'color_intensity', 'hue', 'od280/od315_of_diluted_wines', 'proline']
Data example: [1.423e+01 1.710e+00 2.430e+00 1.560e+01 1.270e+02 2.800e+00 3.060e+00
2.800e-01 2.290e+00 5.640e+00 1.040e+00 3.920e+00 1.065e+03]
[0 1 2]
[0 1 2]
[46]:
from eland.ml import MLModel
# Serialize the scikit-learn model into Elasticsearch
ed_classifier = MLModel.import_model(
es_client=es,
model_id="wine-classifier",
model=sk_classifier,
feature_names=digits.feature_names,
overwrite=True
)
# Capture the Elasticsearch API call w/ logging
import logging
logger = logging.getLogger("elasticsearch")
logger.setLevel(logging.DEBUG)
logger.addHandler(logging.StreamHandler())
# Use the same data as before, but now with the model in Elasticsearch
print(ed_classifier.predict(digits.data[[10, 80, 140]].tolist()))
print(digits.target[[10, 80, 140]])
logger.handlers = []
POST https://167e473c7bba4bae85004385d4e0ce46.us-central1.gcp.cloud.es.io/_ingest/pipeline/_simulate [status:200 request:0.053s]
> {"pipeline":{"processors":[{"inference":{"model_id":"wine-classifier","inference_config":{"classification":{}},"field_map":{}}}]},"docs":[{"_source":{"alcohol":14.1,"malic_acid":2.16,"ash":2.3,"alcalinity_of_ash":18.0,"magnesium":105.0,"total_phenols":2.95,"flavanoids":3.32,"nonflavanoid_phenols":0.22,"proanthocyanins":2.38,"color_intensity":5.75,"hue":1.25,"od280/od315_of_diluted_wines":3.17,"proline":1510.0}},{"_source":{"alcohol":12.0,"malic_acid":0.92,"ash":2.0,"alcalinity_of_ash":19.0,"magnesium":86.0,"total_phenols":2.42,"flavanoids":2.26,"nonflavanoid_phenols":0.3,"proanthocyanins":1.43,"color_intensity":2.5,"hue":1.38,"od280/od315_of_diluted_wines":3.12,"proline":278.0}},{"_source":{"alcohol":12.93,"malic_acid":2.81,"ash":2.7,"alcalinity_of_ash":21.0,"magnesium":96.0,"total_phenols":1.54,"flavanoids":0.5,"nonflavanoid_phenols":0.53,"proanthocyanins":0.75,"color_intensity":4.6,"hue":0.77,"od280/od315_of_diluted_wines":2.31,"proline":600.0}}]}
< {"docs":[{"doc":{"_index":"_index","_type":"_doc","_id":"_id","_source":{"alcohol":14.1,"alcalinity_of_ash":18.0,"proanthocyanins":2.38,"od280/od315_of_diluted_wines":3.17,"total_phenols":2.95,"magnesium":105.0,"flavanoids":3.32,"proline":1510.0,"malic_acid":2.16,"ash":2.3,"nonflavanoid_phenols":0.22,"hue":1.25,"color_intensity":5.75,"ml":{"inference":{"predicted_value":"0","model_id":"wine-classifier"}}},"_ingest":{"timestamp":"2020-07-08T15:35:49.98965Z"}}},{"doc":{"_index":"_index","_type":"_doc","_id":"_id","_source":{"alcohol":12.0,"alcalinity_of_ash":19.0,"proanthocyanins":1.43,"od280/od315_of_diluted_wines":3.12,"total_phenols":2.42,"magnesium":86.0,"flavanoids":2.26,"proline":278.0,"malic_acid":0.92,"ash":2.0,"nonflavanoid_phenols":0.3,"hue":1.38,"color_intensity":2.5,"ml":{"inference":{"predicted_value":"1","model_id":"wine-classifier"}}},"_ingest":{"timestamp":"2020-07-08T15:35:49.98966Z"}}},{"doc":{"_index":"_index","_type":"_doc","_id":"_id","_source":{"alcohol":12.93,"alcalinity_of_ash":21.0,"proanthocyanins":0.75,"od280/od315_of_diluted_wines":2.31,"total_phenols":1.54,"magnesium":96.0,"flavanoids":0.5,"proline":600.0,"malic_acid":2.81,"ash":2.7,"nonflavanoid_phenols":0.53,"hue":0.77,"color_intensity":4.6,"ml":{"inference":{"predicted_value":"2","model_id":"wine-classifier"}}},"_ingest":{"timestamp":"2020-07-08T15:35:49.989672Z"}}}]}
[0 1 2]
[0 1 2]
[47]:
json({"pipeline":{"processors":[{"inference":{"model_id":"wine-classifier","inference_config":{"classification":{}},"field_map":{}}}]},"docs":[{"_source":{"alcohol":14.1,"malic_acid":2.16,"ash":2.3,"alcalinity_of_ash":18.0,"magnesium":105.0,"total_phenols":2.95,"flavanoids":3.32,"nonflavanoid_phenols":0.22,"proanthocyanins":2.38,"color_intensity":5.75,"hue":1.25,"od280/od315_of_diluted_wines":3.17,"proline":1510.0}},{"_source":{"alcohol":12.0,"malic_acid":0.92,"ash":2.0,"alcalinity_of_ash":19.0,"magnesium":86.0,"total_phenols":2.42,"flavanoids":2.26,"nonflavanoid_phenols":0.3,"proanthocyanins":1.43,"color_intensity":2.5,"hue":1.38,"od280/od315_of_diluted_wines":3.12,"proline":278.0}},{"_source":{"alcohol":12.93,"malic_acid":2.81,"ash":2.7,"alcalinity_of_ash":21.0,"magnesium":96.0,"total_phenols":1.54,"flavanoids":0.5,"nonflavanoid_phenols":0.53,"proanthocyanins":0.75,"color_intensity":4.6,"hue":0.77,"od280/od315_of_diluted_wines":2.31,"proline":600.0}}]})
{
"docs": [
{
"_source": {
"alcalinity_of_ash": 18.0,
"alcohol": 14.1,
"ash": 2.3,
"color_intensity": 5.75,
"flavanoids": 3.32,
"hue": 1.25,
"magnesium": 105.0,
"malic_acid": 2.16,
"nonflavanoid_phenols": 0.22,
"od280/od315_of_diluted_wines": 3.17,
"proanthocyanins": 2.38,
"proline": 1510.0,
"total_phenols": 2.95
}
},
{
"_source": {
"alcalinity_of_ash": 19.0,
"alcohol": 12.0,
"ash": 2.0,
"color_intensity": 2.5,
"flavanoids": 2.26,
"hue": 1.38,
"magnesium": 86.0,
"malic_acid": 0.92,
"nonflavanoid_phenols": 0.3,
"od280/od315_of_diluted_wines": 3.12,
"proanthocyanins": 1.43,
"proline": 278.0,
"total_phenols": 2.42
}
},
{
"_source": {
"alcalinity_of_ash": 21.0,
"alcohol": 12.93,
"ash": 2.7,
"color_intensity": 4.6,
"flavanoids": 0.5,
"hue": 0.77,
"magnesium": 96.0,
"malic_acid": 2.81,
"nonflavanoid_phenols": 0.53,
"od280/od315_of_diluted_wines": 2.31,
"proanthocyanins": 0.75,
"proline": 600.0,
"total_phenols": 1.54
}
}
],
"pipeline": {
"processors": [
{
"inference": {
"field_map": {},
"inference_config": {
"classification": {}
},
"model_id": "wine-classifier"
}
}
]
}
}
[48]:
json({"docs":[{"doc":{"_index":"_index","_type":"_doc","_id":"_id","_source":{"alcohol":14.1,"alcalinity_of_ash":18.0,"proanthocyanins":2.38,"od280/od315_of_diluted_wines":3.17,"total_phenols":2.95,"magnesium":105.0,"flavanoids":3.32,"proline":1510.0,"malic_acid":2.16,"ash":2.3,"nonflavanoid_phenols":0.22,"hue":1.25,"color_intensity":5.75,"ml":{"inference":{"predicted_value":"0","model_id":"wine-classifier"}}},"_ingest":{"timestamp":"2020-07-08T15:35:49.98965Z"}}},{"doc":{"_index":"_index","_type":"_doc","_id":"_id","_source":{"alcohol":12.0,"alcalinity_of_ash":19.0,"proanthocyanins":1.43,"od280/od315_of_diluted_wines":3.12,"total_phenols":2.42,"magnesium":86.0,"flavanoids":2.26,"proline":278.0,"malic_acid":0.92,"ash":2.0,"nonflavanoid_phenols":0.3,"hue":1.38,"color_intensity":2.5,"ml":{"inference":{"predicted_value":"1","model_id":"wine-classifier"}}},"_ingest":{"timestamp":"2020-07-08T15:35:49.98966Z"}}},{"doc":{"_index":"_index","_type":"_doc","_id":"_id","_source":{"alcohol":12.93,"alcalinity_of_ash":21.0,"proanthocyanins":0.75,"od280/od315_of_diluted_wines":2.31,"total_phenols":1.54,"magnesium":96.0,"flavanoids":0.5,"proline":600.0,"malic_acid":2.81,"ash":2.7,"nonflavanoid_phenols":0.53,"hue":0.77,"color_intensity":4.6,"ml":{"inference":{"predicted_value":"2","model_id":"wine-classifier"}}},"_ingest":{"timestamp":"2020-07-08T15:35:49.989672Z"}}}]})
{
"docs": [
{
"doc": {
"_id": "_id",
"_index": "_index",
"_ingest": {
"timestamp": "2020-07-08T15:35:49.98965Z"
},
"_source": {
"alcalinity_of_ash": 18.0,
"alcohol": 14.1,
"ash": 2.3,
"color_intensity": 5.75,
"flavanoids": 3.32,
"hue": 1.25,
"magnesium": 105.0,
"malic_acid": 2.16,
"ml": {
"inference": {
"model_id": "wine-classifier",
"predicted_value": "0"
}
},
"nonflavanoid_phenols": 0.22,
"od280/od315_of_diluted_wines": 3.17,
"proanthocyanins": 2.38,
"proline": 1510.0,
"total_phenols": 2.95
},
"_type": "_doc"
}
},
{
"doc": {
"_id": "_id",
"_index": "_index",
"_ingest": {
"timestamp": "2020-07-08T15:35:49.98966Z"
},
"_source": {
"alcalinity_of_ash": 19.0,
"alcohol": 12.0,
"ash": 2.0,
"color_intensity": 2.5,
"flavanoids": 2.26,
"hue": 1.38,
"magnesium": 86.0,
"malic_acid": 0.92,
"ml": {
"inference": {
"model_id": "wine-classifier",
"predicted_value": "1"
}
},
"nonflavanoid_phenols": 0.3,
"od280/od315_of_diluted_wines": 3.12,
"proanthocyanins": 1.43,
"proline": 278.0,
"total_phenols": 2.42
},
"_type": "_doc"
}
},
{
"doc": {
"_id": "_id",
"_index": "_index",
"_ingest": {
"timestamp": "2020-07-08T15:35:49.989672Z"
},
"_source": {
"alcalinity_of_ash": 21.0,
"alcohol": 12.93,
"ash": 2.7,
"color_intensity": 4.6,
"flavanoids": 0.5,
"hue": 0.77,
"magnesium": 96.0,
"malic_acid": 2.81,
"ml": {
"inference": {
"model_id": "wine-classifier",
"predicted_value": "2"
}
},
"nonflavanoid_phenols": 0.53,
"od280/od315_of_diluted_wines": 2.31,
"proanthocyanins": 0.75,
"proline": 600.0,
"total_phenols": 1.54
},
"_type": "_doc"
}
}
]
}
[50]:
print(df[df["zipcode"] > df["score"]].es_info())
es_index_pattern: nyc-restaurants
Index:
es_index_field: _id
is_source_field: False
Mappings:
capabilities:
es_field_name is_source es_dtype es_date_format pd_dtype is_searchable is_aggregatable is_scripted aggregatable_es_field_name
camis camis True long None int64 True True False camis
dba dba True text None object True False False None
boro boro True keyword None object True True False boro
building building True keyword None object True True False building
street street True keyword None object True True False street
zipcode zipcode True short None int64 True True False zipcode
phone phone True keyword None object True True False phone
cuisine_description cuisine_description True keyword None object True True False cuisine_description
inspection_date inspection_date True keyword None object True True False inspection_date
action action True keyword None object True True False action
violation_code violation_code True keyword None object True True False violation_code
violation_description violation_description True keyword None object True True False violation_description
critical_flag critical_flag True keyword None object True True False critical_flag
score score True double None float64 True True False score
grade grade True keyword None object True True False grade
grade_date grade_date True keyword None object True True False grade_date
record_date record_date True keyword None object True True False record_date
inspection_type inspection_type True keyword None object True True False inspection_type
community_board community_board True double None float64 True True False community_board
council_district council_district True double None float64 True True False council_district
census_tract census_tract True double None float64 True True False census_tract
bin bin True double None float64 True True False bin
bbl bbl True double None float64 True True False bbl
nta nta True keyword None object True True False nta
location location True geo_point None object True True False location
Operations:
tasks: [('boolean_filter': ('boolean_filter': {'script': {'script': {'source': "doc['zipcode'].value > doc['score'].value", 'lang': 'painless'}}}))]
size: None
sort_params: None
_source: ['camis', 'dba', 'boro', 'building', 'street', 'zipcode', 'phone', 'cuisine_description', 'inspection_date', 'action', 'violation_code', 'violation_description', 'critical_flag', 'score', 'grade', 'grade_date', 'record_date', 'inspection_type', 'community_board', 'council_district', 'census_tract', 'bin', 'bbl', 'nta', 'location']
body: {'query': {'script': {'script': {'source': "doc['zipcode'].value > doc['score'].value", 'lang': 'painless'}}}}
post_processing: []
[ ]: